Create a predictive model to predict whether a given song's danceability based on its musical features
Data Science Group Project
Dancing is a universal form of art that can be performed by anyone. Be it ballet, hip hop or even 30 second TikTok dances, the majority of us have participated in some form of dancing. Often, most dances are accompanied by music. Hence, we wanted to explore the relationship between dance and music.
The position of Spotify as the reigning audio streaming service provider has remained undisputed throughout recent years, with over 50 million songs listed on their platform and 271 million total monthly active users. Quoting from Spotify's Chief Marketing Officer Seth Farbman, “There has been some debate about whether big data is muting creativity in marketing, but we have turned that on its head. For us, data inspires and gives an insight into the emotion that people are expressing.". Evidently, Spotify’s services are highly data-driven. Therefore, we chose Spotify as our source of data.
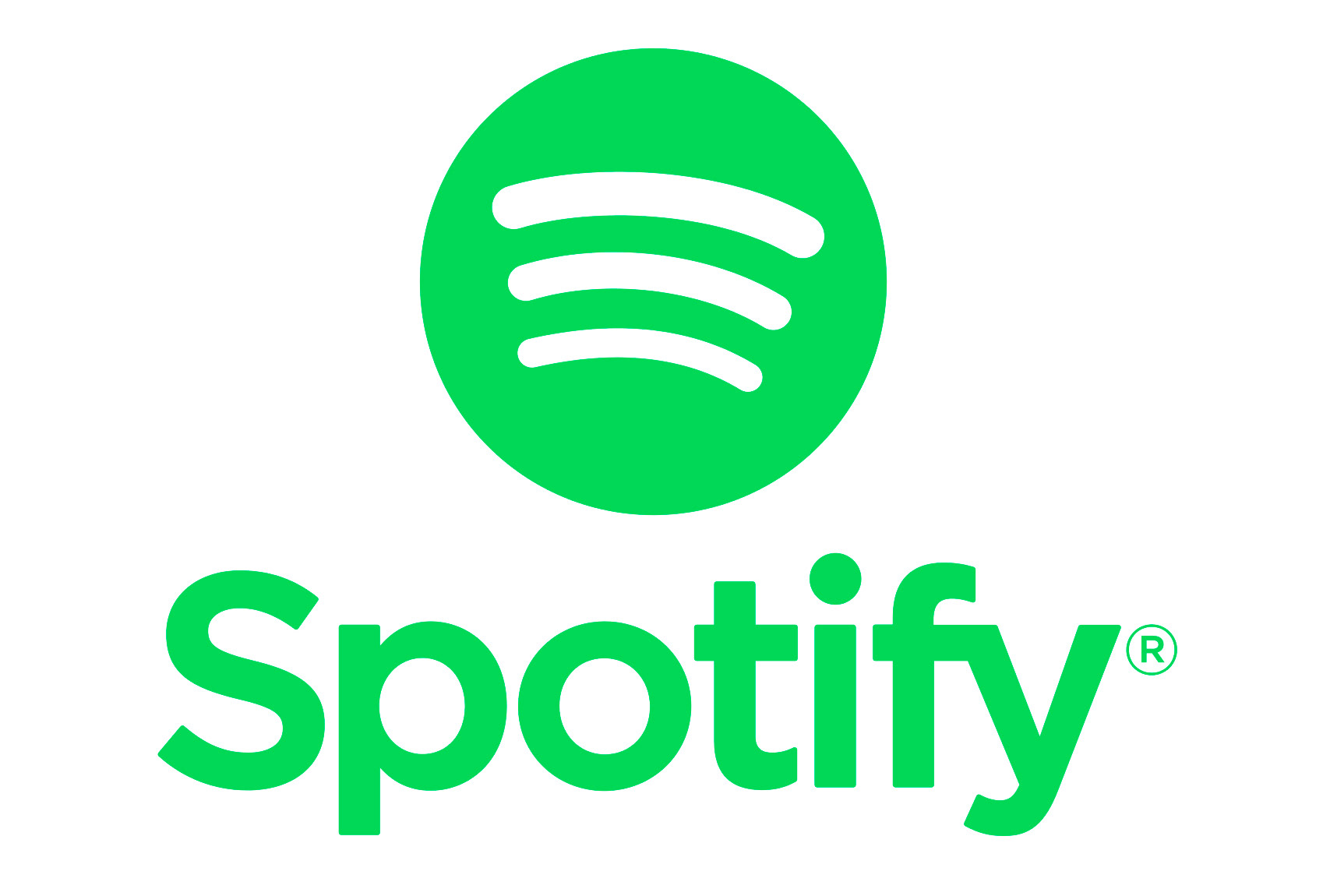
Exploratory Analysis to Understand the Dataset
Exploratory analysis is used to understand our dataset - to identify the most relevant features.
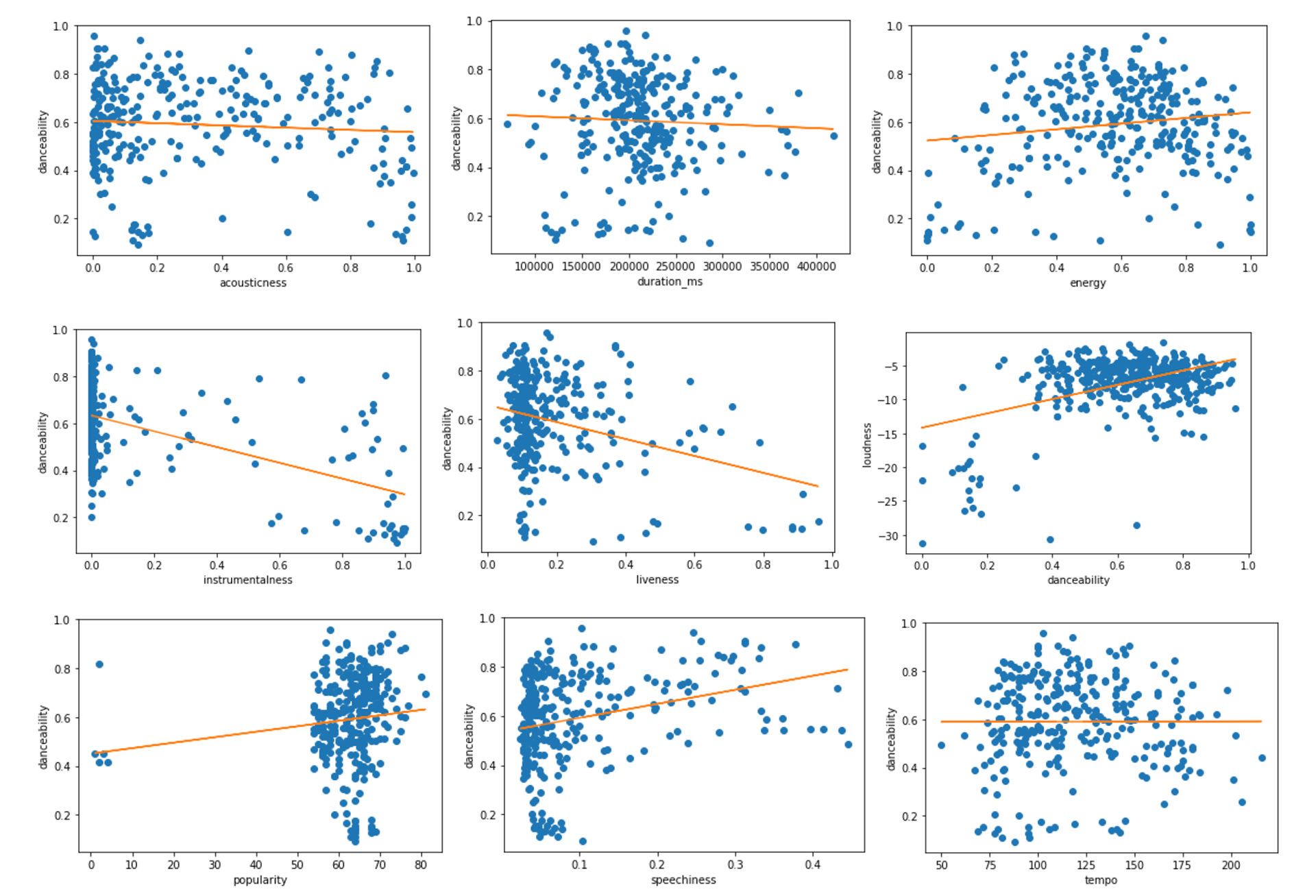
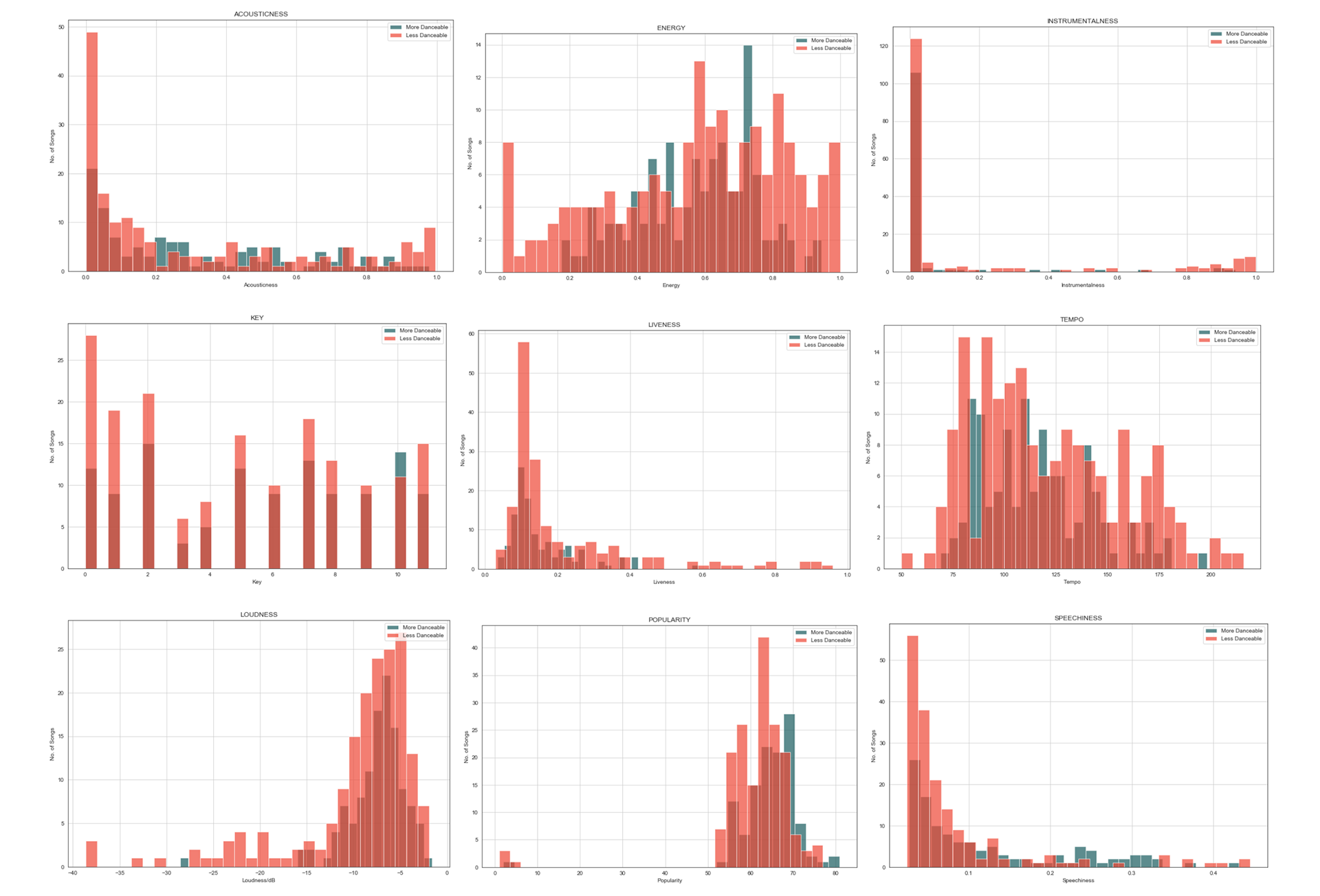
Examples of Predictive Model
Various predictive models were built, including logistic regression, decision tree, random forest and support vector machine. Finally, the logistic regression model was selected as it performed the best in terms of accuracy and precision. It achieved an accuracy of 67.8%.
By training and enhancing our model with further newly released song data, its accuracy and precision will improve, thus increasing its capability of picking the best songs for dancing.
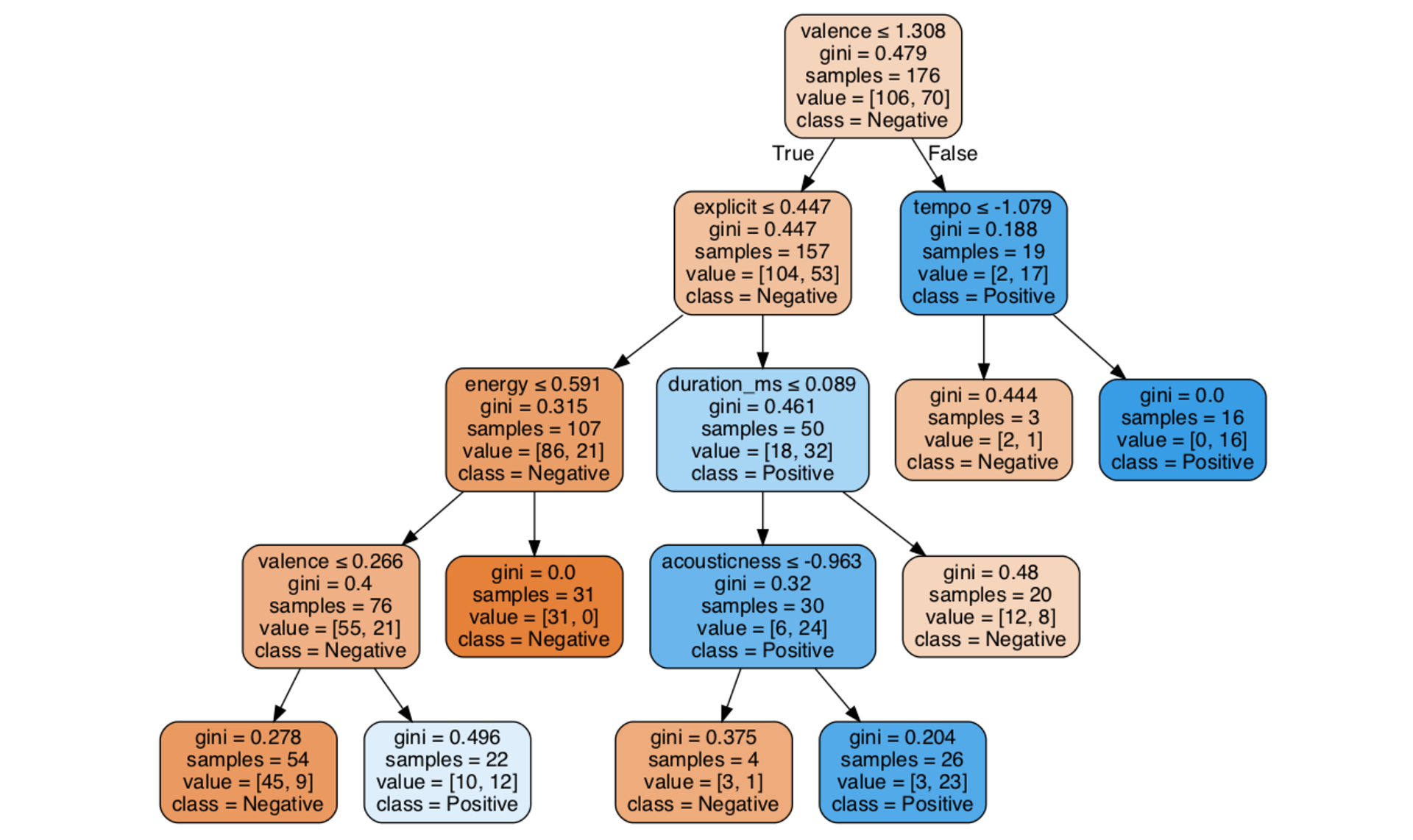
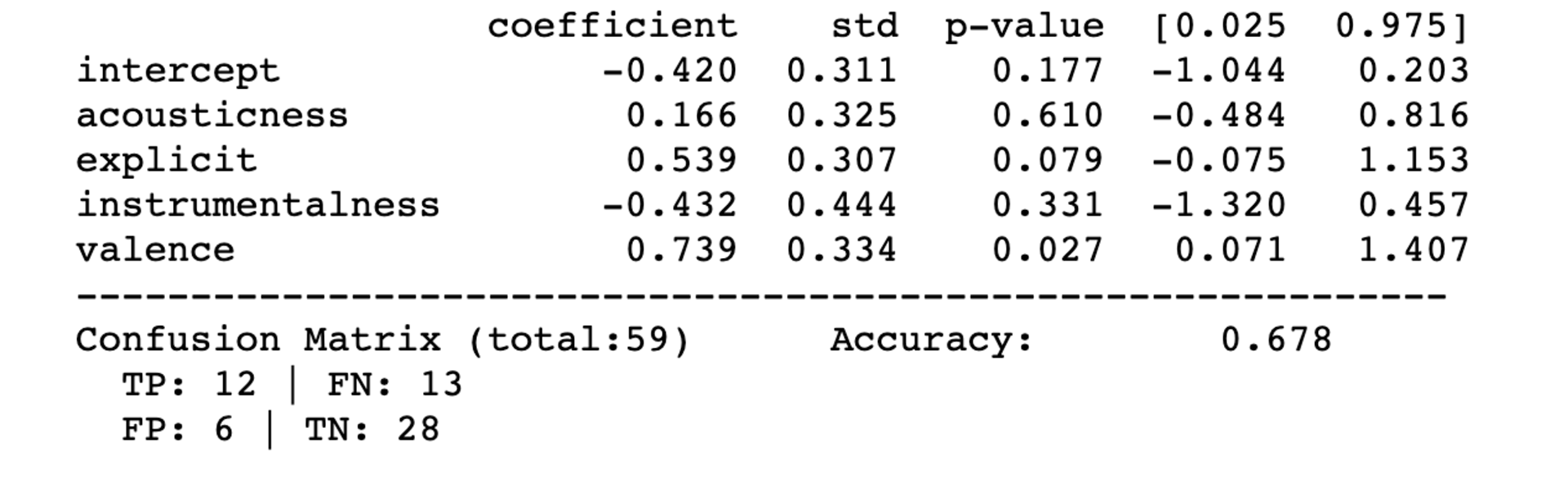